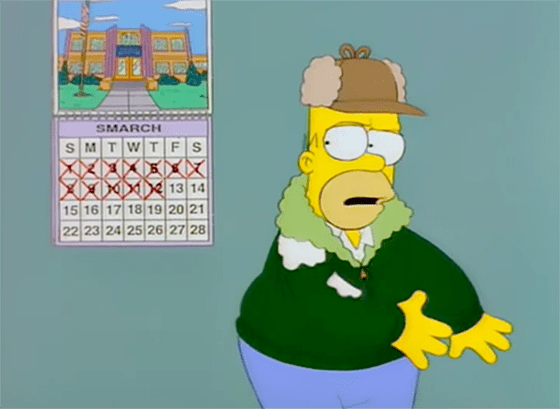
For many marketers, the biggest barrier to running A/B tests is time.
As a fellow busy marketer, I get it. We’ve all got a bottomless inbox, an ever-growing day-to-day task list and bigger projects to tackle.
But I also know that 60 minutes of planning each month is all it takes to get you out of your habit of A/B testing procrastination.
A/B testing calendars are a plan for your team detailing what tests you’re going to run each week or month, what they aim to optimize and when they start and finish.
They motivate you to keep lining up tests, but they also help you become more efficient so you can generate more tests (and more wins). Check out the graph below, which shows the number of tests per month I was able to run before and after implementing an A/B testing calendar:
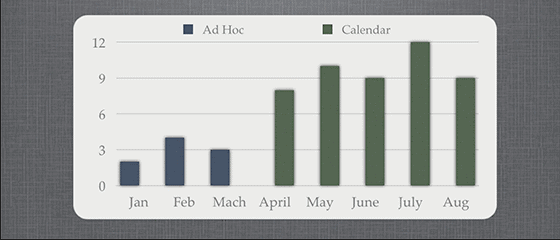
Needles to say, the extra conversion rate uplift you can gain from running twice as many tests can be enormous.
If you’re ready to get out of your procrastination funk, then read on. This post will help you implement a solid A/B testing calendar so you can finally become an A/B testing veteran who sees consistent conversion uplifts month after month.
Step 1: Know your tests
The first step is to choose what you’d like to optimize.
There are many places you can start, from your landing pages to your user acquisition and onboarding flows.
The trick is to just pick one and get started. For example:
- Test your homepage using tools like Optimizely or VWO.
- Test your campaign landing pages using Unbounce.
- Test your onboarding flow (and onboarding emails) with services like SparkPage or Vero.
Listing these areas out is an important first step. Once you’ve chosen a larger area to test, you can get a little more granular and formulate your first hypothesis.
The guys at ConversionXL have a great guide to help you formulate and prioritize killer hypotheses. Here’s a quick summary:
- Identify problem areas. Use your analytics to find high-traffic pages with high drop-off rates.
- Interview real users. Use insight and data together to come up with better test hypotheses.
- Prioritize your tests. Rank them based on three criteria: the potential impact on a certain metric, the importance of that metric and the cost to implement.
Step 2: Count your conversions per month
Next, you’ll need to take a look at past tests and estimate how many conversions per month you can get in each area.
Your homepage, for example, likely gets the most traffic and therefore gets the most conversions per month.
Additionally, we’ll start with a rule of thumb that you’ll want about 250 conversions per variant to reach statistical significance. This is a good figure to get you started, but check out Optimizely’s sample size calculator to work out a more exact figure.
With these two numbers, (average conversions per month and number of conversions to reach statistical significance), you can calculate your the number of variants you can test for that month.
Calculating number of variants per month
For example, lets imagine you have one landing page for your latest ebook, which gets 5,000 visitors per month and a 15% conversion rate. This means 750 ebook downloads (conversions) per month.
So using our 250 conversions “rule of thumb,” you could test 3 variants per month (750/250).
Step 3: Standardize to one time period
After you do that simple calculation, you’ll know how many variants you can run per month in each testing area.
This is where the real value to this method comes in.
When you run tests in a non-standardized way, your testing calendar might look something like this:
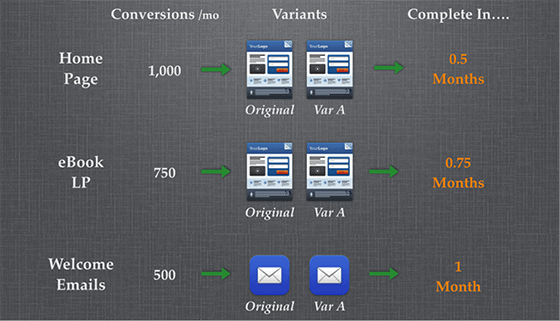
Because no tests have a definite time length, all your tests start and finish at different times, which is incredibly difficult to plan around.
Now imagine you plan each test so that it runs for exactly a month, incorporating the max number of variants that the conversions allow. Here’s what that’d look like:
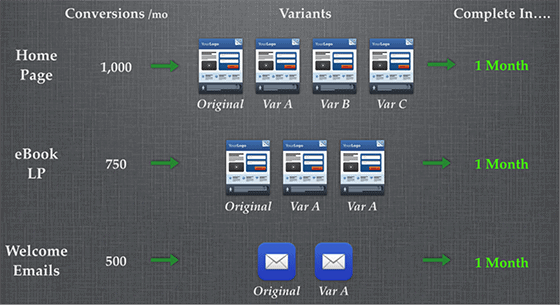
This is much, much easier to plan your testing calendar around, as you can to start and end on the same day.
If you shift to planning tests that run for a specific time period, your monthly team meetings will start to look like this:
- All the tests running last month have finished and you have the results ready for the team to discuss and share lessons learned.
- The tests for this month are already underway.
- You spend some time brainstorming and planning the test for next month.
Your team now finishes each test at the end of the month and on the same day, they can start the next test.
There is no time wasted between the end of one test and the start of another. Every possible hour that you could be testing, you are testing.
You are now an unstoppable optimization machine. :)
Step 4: Take the template, make it work for you
Here is the template we use each month. It’s yours to take, download and modify.
There is space to identify each test, set the hypothesis and define the goal. (If you haven’t done this before, you might want to read “How to Formulate A Smart A/B Test Hypothesis.”)
We have a separate tab for each of our test areas, then a month-by-month plan for each channel. If you have enough resources and traffic to run tests weekly or bi-weekly, then just change “Month 1” to “Week 1.”
And if you want to dig even deeper into A/B test objective and goal setting, check out this webinar from Optimizely and KISSmetrics. They discuss A/B test planning at 32:00.
Your next action: Take 15 minutes
It doesn’t take much to get started on this. In fact, you can get started with your A/B testing calendar in 15 minutes flat.
- First, map the key areas in your user acquisition journey. For example, your homepage, campaign landing pages, onboarding flow and welcome emails.
- Next, download our template and create a new tab for each area you’ve identified. Change the timeframe in each (month, week, etc) to match your testing schedule.
- List any tests you currently have running and suggest a test in each area for next month.
- Between now and the start of next month, flesh out the details of each test. Determine the variants you want to test and ready any assets you’ll need, like copy and images.
And lastly, if you have any other advice to share on how your team manages your A/B testing calendar, please leave a comment and let us know!